Efficient Local RL Fine-Tuning of Reasoning Models
Fine-tuning large language models (LLMs) to improve their reasoning abilities typically requires extensive computational resources. However, efficient local reinforcement learning (RL) techniques, such as Low-Rank Adaptation (LoRA), offer a practical solution. LoRA achieves this efficiency by updating only a small number of parameters through low-rank matrix approximations, significantly reducing computational demands without sacrificing performance. This targeted approach enables faster adaptation of LLMs for specific reasoning tasks, making advanced capabilities accessible even with limited resources.
Reasoning models enhanced through this approach demonstrate improved logical consistency and decision-making in tasks like problem-solving, planning, and complex question-answering. By combining LoRA with RL, models can adapt based on performance-driven feedback, efficiently learning from fewer examples. This synergy allows under-resourced academic or research environments to fine-tune powerful reasoning models effectively, broadening access to cutting-edge AI capabilities.
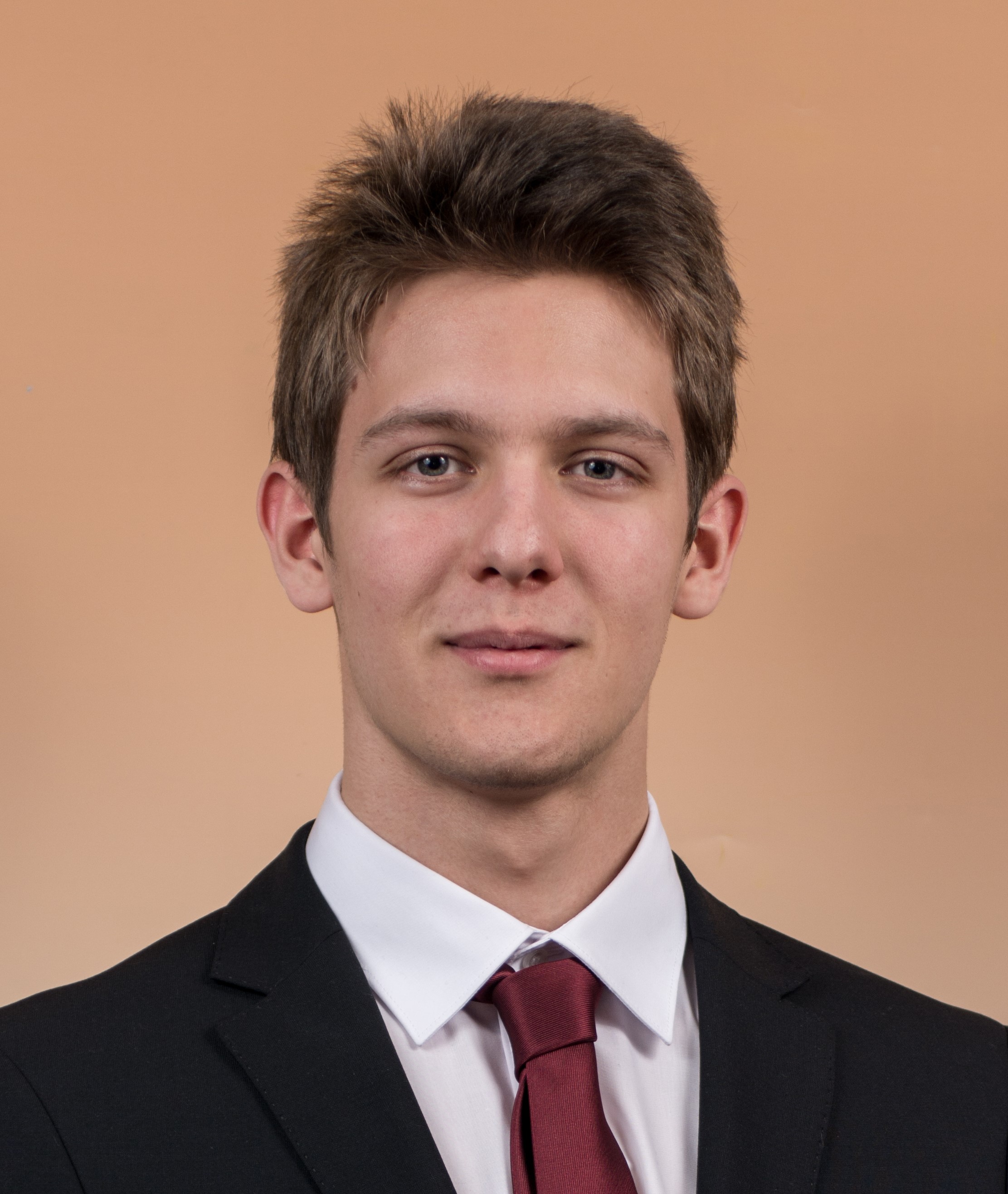
Antal Mátyás
doktorandusz
antal.matyas (*) mit * bme * hu