Embedded Artificial Intelligence Laboratory
VIMIMB05 | Electrical Engineering MSc | Semester: 3 | Credit: 5
Objectives, learning outcomes and obtained knowledge
The laboratory aims to apply the knowledge acquired in the course Embedded Artificial Intelligence in practice. The primary focus of the laboratory is for students to be able to independently apply artificial intelligence methods to real physical measurements and data sets, and to gain practical experience of their advantages and limitations. We implement the algorithms on embedded platforms.
Lecturers
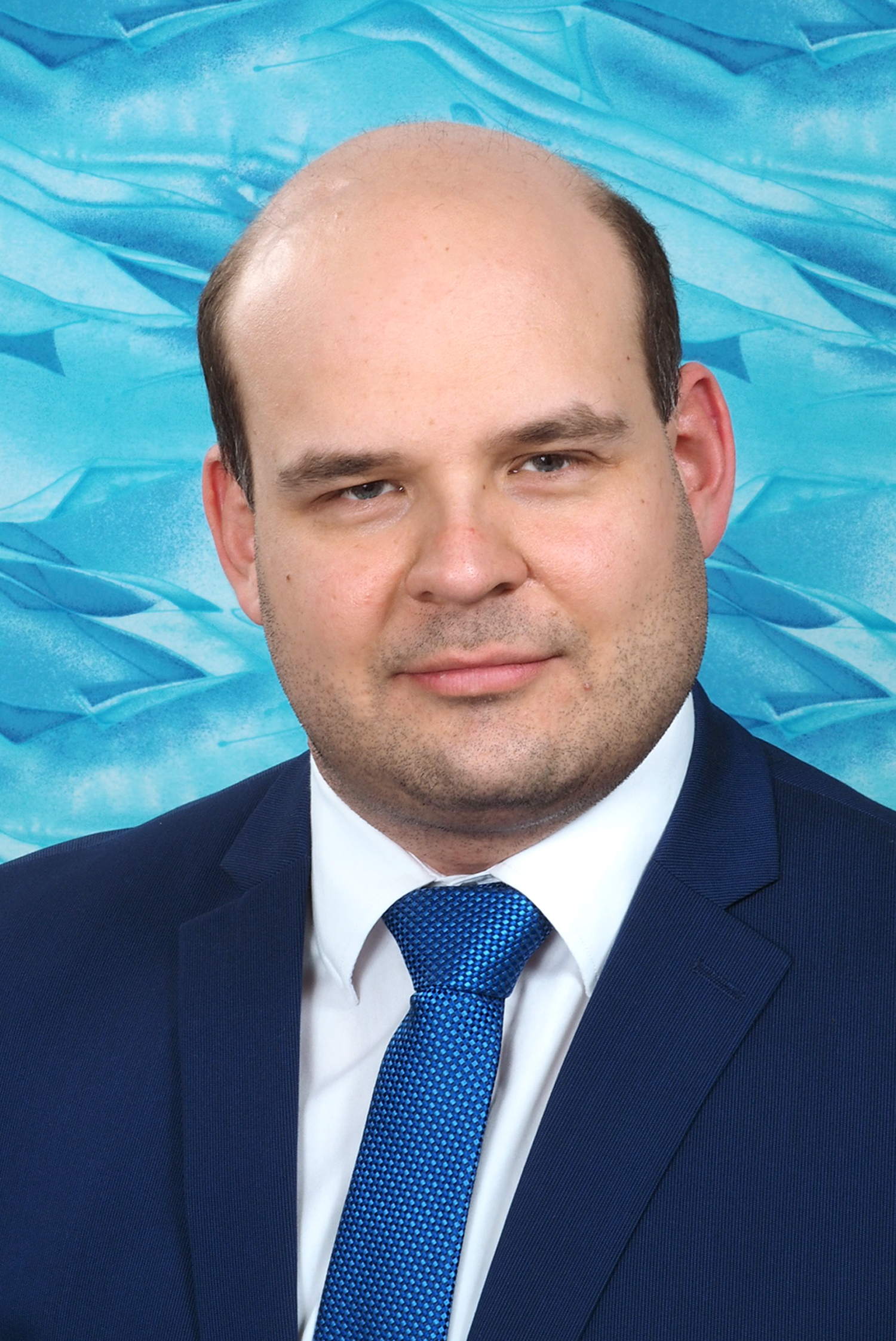
Barna Csuka
assistant professor
Course coordinator
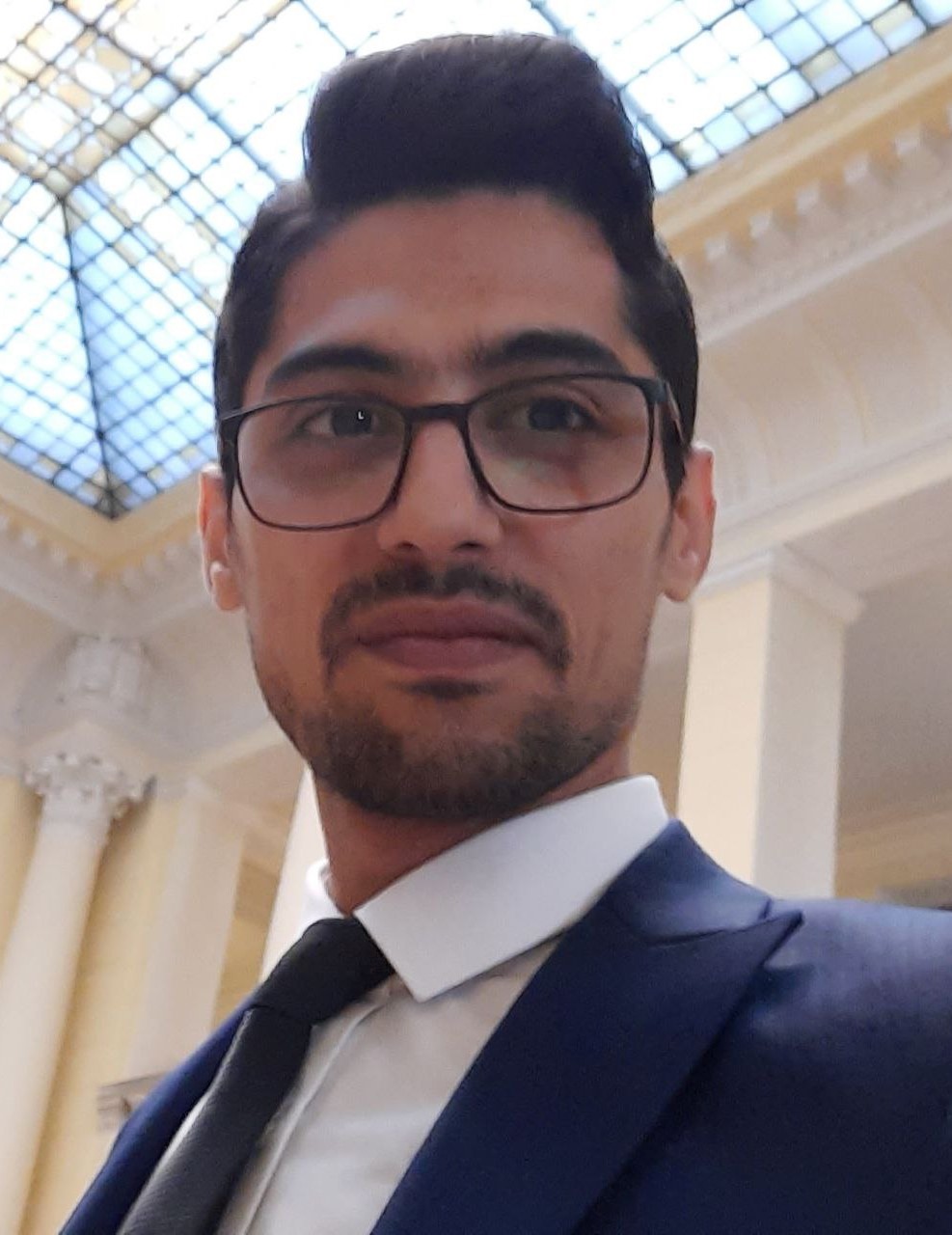
Hussein Al-Rikabi
PhD student
Synopsis
- Gradient-based investigation of the error backpropagation algorithm of neural networks in an embedded environment, parameterization of the learning factor based on the moment, batch sizes and instantaneous slope.
- The effect of different activation functions on the speed of learning and the performance of the model. Examination of the robustness of neural network models using dropout and ablation methods.
- Examining the bias-variance dilemma in machine learning methods. Identification of the noise component of the training data set and its effect on the best available model performances.
- GPU-based acceleration of neural network architectures, their aspects for both training and query use. Performing matrix operations on SIMD architectures, limitations of parallelizability.
- Teaching convolutional neural networks, increasing model robustness with an augmented (shifted/noisy/rotated/mirrored) sample set.
- Implementation of a classification system with multilevel processing, classification with a neural network and fuzzy approach.
- Design of applied joint time and frequency transformations in a hardware environment. Processing of periodic signals, extraction of main parameters.
- Data analysis workflow (outlier detection, data cleaning, handling incomplete data, knowledge modeling). Examination of the physical measurement ranges of modern embedded sensor platforms (smart, wearable devices).
- Application of Kálmán filters to implement offline sensor fusion. Analysis of the applicability of location and position determination by integrating the gyroscope, magnetometer and accelerometer.
- Possibilities and limitations of implementing real-time sensor fusion on embedded systems. The relationship between model complexity and consumption, examination of computing power available on some hardware architectures.