Embedded Artificial Intelligence
VIMIMA22 | Electrical Engineering MSc | Semester: 2 | Credit: 5
Objectives, learning outcomes and obtained knowledge
This course introduces artificial intelligence algorithms for information processing in embedded systems. The speciality is that information is basically data derived from physical processes, and the implementation of the algorithms will be specifically addressed in the context of the realization on embedded platforms.
Lecturers
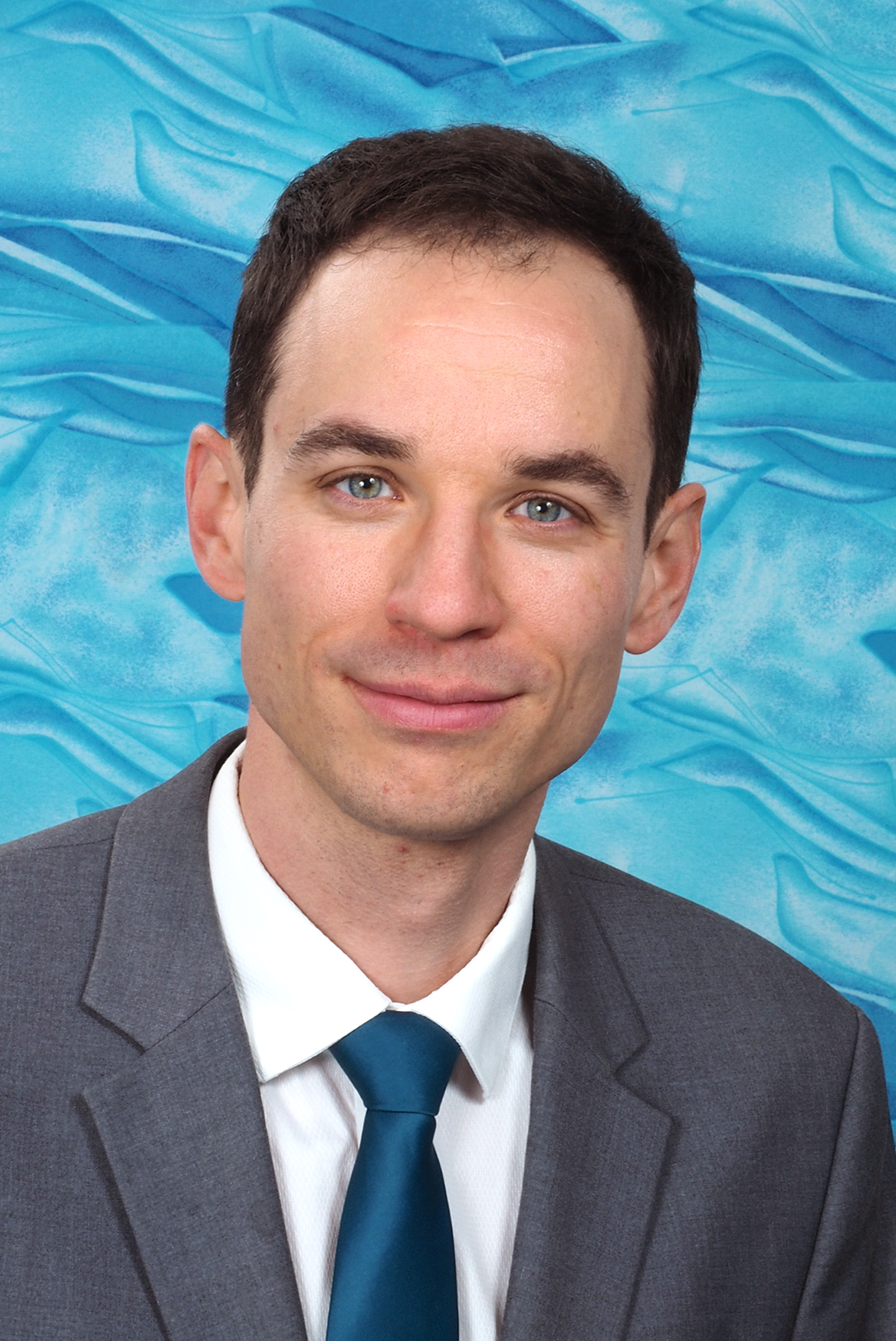
Balázs Renczes
associate professor
Course coordinator
Synopsis
1. Introduction, description of the subject requirements. Overview of the areas of artificial intelligence, its application in embedded systems and the focus of the subject.
2. Description of the data analysis workflow. Outlier detection and data cleaning, handling missing data, exploring the possibilities of knowledge modeling.
3. Analysis of the problems and solutions of regression and classification in a hardware environment, introduction to the related linear and logistic models.
4. Examination of the clustering problem, study of dimensionality reduction options.
5. Introduction to artificial intelligence sensor fusion methods for embedded applications.
6. Introduction to neural networks. Demonstration the effect of noise on the learning process. Examining the problems of overlearning, early stopping and backtracking on different platforms. Decomposition of the sample set into training, test and validation sets.
7. Description of the functionality of convolutional neural networks. Presentation of a pattern recognition system that can be run in an embedded environment.
8. Study of feedback neural networks. Introduction to the possibilities of prediction.
9. Interpreting the output of neurons. Demonstration of the importance of representation learning, description of autoencoder.
10. Overview of application limitations of general purpose devices (microcontroller, FPGA, general purpose processor).
11. Presentation of target hardware for implementing artificial intelligence on embedded platforms.
12. Presentation of smart devices, smart watches capabilities for embedded AI.
1. Application of linear and logistic regression and classification in an embedded environment, using examples with known physical models, testing the representational capabilities of linear models, adding new variables to the model.
2. Challenges of high dimensionality data, removing linear dependencies, applying principal component analysis and singular value decomposition to dimensionality reduction on an embedded platform, quantifying information loss, testing reversibility.
3. Sensor data integration, noise management, measurements from different sources, fusion of different measurement methods in hardware implementation.
4. Implementing applied neural networks in embedded systems, investigating the impact of noise on learning, calculating confidence of convergence, and discussing coupled over-learning, early shutdown and backtracking challenges. Decomposition of samples into training, test and validation sets.
5. Embedded application examples of convolutional neural networks, impact of kernel sizes on representability, explanatory analysis of learned feature vectors.
6. Time-series data analysis on embedded platforms, comparative analysis of autoregressive (ARIMA) methods and feedback neural network-based prediction architectures.
7. Unsupervised feature vector learning, the impact of latent dimensionality on the representativeness of models, sampling of generative models.